
The major aim of the research work is to prepare a shallow parser for Malayalam to enable machine translation, especially between Malayalam and Tamil. For ensuring the quality of the output, an IA agreement has been conducted. Both the taggers, CRF++ (94.39 and 88.87) and SVM (96.85 and 93.59), have outperformed the existing Odia POS taggers in terms of both reliability and accuracy. Finally, the taggers are made online using JSP and JST technology. After identifying and discussing issues, different solutions have been proposed: formulation of linguistic rules, corpus-driven, word sense disambiguation, and application of external tools like NER, WSD, morph analyser.
#ONLINE POS TAGGER MANUAL#
A comprehensive error analysis has been conducted to figure out the types of errors committed by both in common based on which 5-fold manual error correction and final evaluation have been conducted. Evaluation has been conducted on the precision and recall measures for CRF and known-unknown words accuracy for SVM. So far as the experimental set up is concerned, similar feature has been selected to train both the models. For annotating the whole ILCI corpus the BIS annotation scheme has been taken into consideration with some modifications.
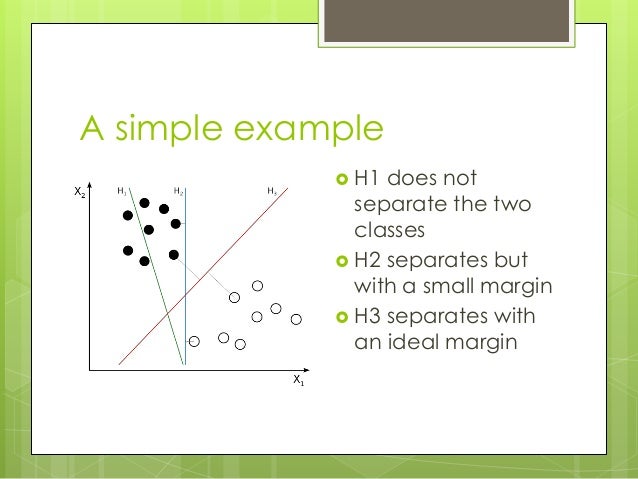
Approximately, 400k tokens have been applied to develop both of them with the training and testing data estimating to 236k and 123k tokens respectively. This study focusses on developing statistical POS taggers for Odia using two distinct algorithms CRF (probability) and SVM (classifier).
